MnModel Phase 4 Methods
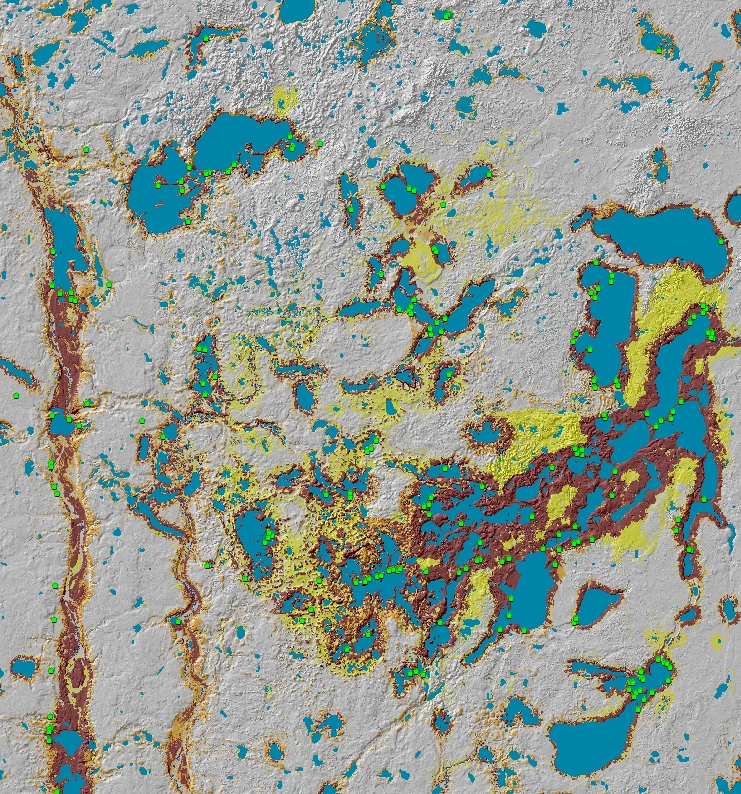
Surface hydrography model
Phase 4 predictive modeling was preceded by the development of data and models of historic and prehistoric environments. Variables based on these environmental models and on updated statistical procedures were key components of the model updates.
Historic/Prehistoric Environmental Models
In Phase 3 the assumption was made that contemporary features could serve as surrogates for features from the past. This was necessary since only modern environmental data were available. For Phase 4, every attempt was made to develop environmental models that better reflected conditions when archaeological artifacts were deposited. These models included:
- Digital Terrain Model (DTM) conditioned to reduce the effects of modern infrastructure, to restore historic terrain in part of the Mesabi iron mining region, and to incorporate bathymetric data beneath reservoir lakes.
- Historic Vegetation Model based on statistical modeling of the observations of General Land Office surveyor’s from their line notes and plat maps.
- Historic/Prehistoric Hydrographic Models to model the distribution and extent of lakes, rivers, and wetlands prior to extensive draining of wetlands and damming of rivers.
- Statewide Landscape Model to assemble the best available geomorphic data for each part of Minnesota and combine these into statewide map with a consistent and meaningful classification system.
Phase 4 Variables
Phase 4 variables were derived primarily from the models described above. Measures of distance to key resources were calculated using Least-Cost Path procedures rather than Euclidean Distance as used in Phase 3. Sampling procedures were developed to represent the mean or majority value of variables within site polygons, instead of the single value at the polygon’s centroid.
Phase 4 Statistical Methods
A 2007 study developed enhanced statistical procedures for MnModel. Its objectives were to find the best prediction method for MnModel Phase 4 that can be implemented reasonably within GIS and to produce S-Plus routines to implement this prediction method.
The project compared eight prediction methods. Of these, bagged trees (bagging) provided the best prediction with the test data. In 2018 we updated these methods to make use of open-source R statistical software and to implement Random Forest, an improved bagging technique.
All GIS tools, SPlus scripts, and R scripts developed for MnModel Phase 4 can be made available to researchers upon request.