Quick Links
Chapters
- Management Summary
- Research Design & Time Line
- Environment & Native American Culture
- GIS Design
- Archaeological Database
- Archaeological & Environmental Variables
- Model Development & Evaluation
- Model Results & Interpretation
- Project Applications
- Model Enhancements
- Model Implementation
- Landscape Suitability Models
- Summary & Recommendations
Appendices
- Archaeological Predictive Modeling: An Overview
- GIS Standards & Procedures
- Archaeology Field Survey Standards, Procedures & Rationale
- Archaeology Field Survey Results
- Geomorphology Survey Profiles, Sections, & Lists
- Building a Macrophysical Climate Model for the State of Minnesota
- Correspondence of Support for Mn/Model
- Glossary
- List of Figures
- List of Tables
- Acknowledgments
Chapter 8
Model Results and Interpretations
By Elizabeth Hobbs, Craig M. Johnson, Guy E. Gibbon, Carol Sersland, Mark Ellis, and Tatiana Nawrocki
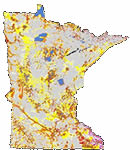
Chapter
8 Table of Contents
8.1 Introduction
8.2 Model Description
8.2.1
Environmental Context
8.2.2
Types of Models
8.3 Model Evaluation
8.4 Model Interpretation
8.4.1
Presentation of Interpretations
8.4.2
Previously Identified Variables
8.5 Model Comparison
8.5.1
Subsection Group Approach
8.5.2
Site Catchment Analysis
8.5.3
Cultural Context and Site Location
8.5.4
SHPO Intuitive Model
8.6 Model Results
8.6.1
Phase 1 Results
8.6.2
Phase 2 Results
8.6.3
Phase 3 Results
8.7 Agassiz Lowlands
8.8 Anoka Sand Plain
8.9 Aspen Parklands
8.10 Big Woods
8.11 Blufflands
8.12 Border Lakes
8.13 Chippewa Plains
8.14 Coteau Moraines / Inner Coteau
8.15 Glacial Lake Superior Plain/Northshore highlands/ Nashwauk
Uplands
8.16 Hardwood Hills
8.17 Laurentian Highlands
8.18 Littlefork-Vermilion Uplands
8.19 Mille Lacs Uplands
8.20 Minnesota River Prairie
8.21 Oak Savanna
8.22 Pine Moraines & Outwash Plains
8.23 Red River Prairie
8.24 Rochester Plateau
8.25 St. Croix Moraines and Outwash Plains (Twin Cities Highlands)
8.26 St. Louis Moraines/ Tamarack Lowlands
8.27 Conclusion
References
In Phase 1 of the project, models for sites excluding single artifacts were developed only for the 29 counties for which "probabilistic" surveys were available (Figure 4.5). These were grouped into five archaeological resource regions (Section 4.6.1) for modeling. Since it was not possible to acquire and convert data for all of these counties in time to meet modeling deadlines, some of the counties were not modeled or were modeled only for one region when they otherwise would have been modeled for two regions. The regions necessarily had incomplete and sometimes discontinuous coverage.
Models were built using only sites from "probabilistic" surveys, excluding single artifacts. All other sites in the counties modeled were used to test the models. Negative survey locations represented non-sites. Modeling methods for this initial phase of the project are discussed in Section 7.3.
Some of these regions were too large and contained too much environmental variability to model well using such small site numbers and environmental data from distant and disjunct areas. For example, when models developed for Nicollet County were applied to all counties in the Prairie Lakes Region, they performed well for counties near Nicollet County but not as well for distant counties. In the Central Lakes Coniferous Region, models performed better for centrally located counties that had more data and less well for counties on the margins of the region, which had fewer sites.
Because of bias in the locations of "probabilistic" surveys (see Chapter 5), there was not a wide range of environmental difference between sites and non-sites. This weakens the models in two ways. First, it reduces the ability of the statistical analysis to distinguish between sites and non-sites. Second, it fails to represent all possible environmental settings, providing potentially fallacious predictions for unsampled landscapes.
In general, site numbers used to build the models were extremely low - only sites from "probabilistic" surveys and then only from some of the counties in each region. Within the modeled areas, only 576 sites were available that met the criteria. However, there is no apparent relationship between the performance of the models and the number of sites used to build them (Table 8.6.1). Site numbers were not, in fact, significantly lower than those used to build individual Phase 2 models excluding single artifacts (Table 8.6.2).
Table 8.6.1. Evaluation of Basic Phase 1 Models, Percent Known Sites (excluding single artifacts) in Each Site Potential Class.
Modeling Region |
# Sites
Modeled |
% Low |
% Medium |
% High |
% High /Medium |
Gain |
Nicollet County (pilot) |
31 |
0 |
14 |
86 |
100 |
0.34 |
Prairie Lakes |
190 |
11 |
18 |
71 |
89 |
0.26 |
Southeast Riverine |
87 |
8 |
13 |
79 |
92 |
0.29 |
Southwest Riverine |
41 |
8 |
17 |
75 |
92 |
0.29 |
Central Lakes Deciduous |
227 |
14 |
27 |
58 |
85 |
0.22 |
Central Lakes Coniferous |
147 |
2 |
13 |
85 |
98 |
0.33 |
Average |
96 |
7 |
17 |
76 |
93 |
0.29 |
Phase 1 models were run using logistic regression in GRID, not S-Plus. This undoubtedly produced weaker models than Phases 2 and 3 for two reasons. First, because there is no stepwise function in GRID for selecting the best model variables, only a limited number of variable combinations could be tried. There is no guarantee that any of these models represents the absolute best set of variables from those available. Second, variable coefficients are rounded in GRID, sometimes to zero for small coefficients. As we found out in Phase 2, small differences in coefficients and attributing zero values to variables with small coefficients can make a considerable difference in model performance.
All Phase 1 models had approximately 33 percent of the landscape classified in each of the low, medium, and high site potential zones (Figure 8.4a). Regional models varied primarily in how many sites were predicted to be in the high site potential zone. These models had gain statistics ranging from 0.22 to 0.34 (Table 8.6.1). Gain statistic values are low because the high and medium probability areas were, by definition, 66 percent of the landscape. The strongest model was developed for a small, homogeneous area (Nicollet County.) The weakest model was for the Central Lakes Deciduous Region (Figure 2.2), where the area modeled was large and the data discontinuous.
Phase 2 models were developed for all 87 counties, divided into 15 modeling regions based on archaeological resource regions (Section 4.6.2). Modeling methods are discussed in Section 7.3. Several variations of site probability models were developed. Basic models refer to those developed using variables that were available statewide. Enhanced models also included variables that were available for only limited parts of the state. Basic and enhanced models were developed for two populations of known sites, one excluding only single artifacts and the other excluding both single artifacts and lithic scatters. A total of 1,815 sites were available statewide that met all modeling criteria and excluded single artifacts. When lithic scatters were removed, this reduced the statewide dataset by 43 percent to 1,048.
The best Phase 2 basic models are summarized in Tables 8.6.2 and 8.6.3. The composite of models excluding only single artifacts is illustrated in Figure 8.4b. A goal set at the beginning of this phase of the project was to develop models with 85 percent of the known sites predicted in 33 percent of the area modeled. That would be the equivalent of a gain statistic of 0.61. Seventy-three percent of the models developed with only single artifacts excluded and 36 percent of those with lithic scatters also excluded met or exceeded this goal. Models excluding only single artifacts produced gains statistics ranging from 0.28 to 0.89, with an average gain of 0.68 (Table 8.6.2). Models excluding both single artifacts and lithic scatters had gains from 0.12 to 0.94, with an average gain of 0.61 (Table 8.6.3).
These results indicate that models excluding only single artifacts performed, on average, much better than models excluding single artifacts and lithic scatters. However, results varied between regions. While gain statistics for some regions may decline from incorporating lithic scatters as part of the database, this loss is less than 0.10 in all but two regions (Table 8.6.4). However, the increased gain from including lithic scatters is less than 0.10 in only one region. On the average, the gain statistic increases by 0.11 from including lithic scatters in the database. Whether the improvement is attributable to the particular information contained in the lithic scatter locations or simply due to the increase in the number of sites modeled could be debated. The Lake Superior model would not run at all when removing lithic scatters reduced the database from eight sites to three. Certainly the inclination is to have more confidence in models built with large numbers of sites. However, all of the site populations modeled in Phase 2 are smaller than ideal for multivariate analysis. The inclusion of lithic scatters could improve models in cases where lithic scatters have similar environmental settings as other modeled sites by enhancing the detectable pattern. On the other hand, if lithic scatters are found in different environmental settings, or if there is no pattern to where they are found, they could degrade model performance by muddling the "pattern." Consequently, it is important to remember that the detectable pattern from such small site populations, no matter how distinct, may not be representative of the entire universe of sites, most of which have not yet been found. Even relatively modest changes in the number and characteristics of the site population may exert a strong influence on model results. Only very large site populations from a random sample of all landscapes in a region will produce a representative database for modeling.
Table 8.6.2. Best Phase 2 Basic Models (excluding single artifacts).
Modeling Region |
# Sites
Modeled |
% Area High/ Medium |
% Sites Predicted |
Gain |
Southwest Riverine |
41 |
35 |
86 |
0.59 |
Prairie Lakes East |
77 |
25 |
64 |
0.61 |
Prairie Lakes North |
120 |
20 |
71 |
0.71 |
Prairie Lakes South |
209 |
20 |
77 |
0.74 |
Southeast Riverine East |
58 |
34 |
72 |
0.53 |
Southeast Riverine West |
63 |
63 |
87 |
0.28 |
Central Lakes Deciduous East |
278 |
18 |
82 |
0.78 |
Central Lakes Deciduous South |
199 |
24 |
81 |
0.70 |
Central Lakes Deciduous West |
165 |
18 |
81 |
0.78 |
Central Lakes Coniferous South and East |
119 |
15 |
78 |
0.81 |
Central Lakes Coniferous Central, North, and West |
236 |
19 |
77 |
0.75 |
Red River Valley |
80 |
19 |
79 |
0.76 |
Northern Bog |
8 |
33 |
59 |
0.44 |
Border Lakes |
154 |
10 |
81 |
0.88 |
Lake Superior |
8 |
8 |
74 |
0.89 |
Average |
121 |
24 |
77 |
0.68 |
Table 8.6.3. Best Phase 2 Basic Models (excluding single artifacts and lithic scatters).
Modeling Region |
# Sites
Modeled |
% Area High/ Medium |
% Sites Predicted |
Gain |
Southwest Riverine |
11 |
80 |
91 |
0.12 |
Prairie Lakes East |
29 |
30 |
56 |
0.46 |
Prairie Lakes North |
74 |
49 |
66 |
0.26 |
Prairie Lakes South |
91 |
40 |
82 |
0.51 |
Southeast Riverine East |
38 |
17 |
62 |
0.73 |
Southeast Riverine West |
54 |
48 |
81 |
0.41 |
Central Lakes Deciduous East |
196 |
10 |
76 |
0.87 |
Central Lakes Deciduous South |
105 |
34 |
80 |
0.58 |
Central Lakes Deciduous West |
103 |
19 |
81 |
0.77 |
Central Lakes Coniferous South and East |
5 |
22 |
43 |
0.49 |
Central Lakes Coniferous Central, North, and West |
171 |
43 |
84 |
0.49 |
Red River Valley |
44 |
15 |
78 |
0.81 |
Northern Bog |
6 |
44 |
81 |
0.46 |
Border Lakes |
118 |
5 |
78 |
0.94 |
Lake Superior |
3 |
NA |
NA |
NA |
Average |
70 |
35 |
80 |
0.61 |
Table 8.6.4. Comparison of Phase 2 Models With and Without Lithic Scatters.
Modeled Region |
Difference
in number of sites modeled (number of lithic scatters) |
Lithic
scatters as percentage of all sites excluding single artifacts |
Gain excluding single artifacts minus gain also excluding lithic scatters |
Southwest Riverine |
30 |
73 |
0.47 |
Prairie Lakes East |
48 |
62 |
0.15 |
Prairie Lakes North |
46 |
38 |
0.45 |
Prairie Lakes South |
118 |
56 |
0.23 |
Southeast Riverine East |
20 |
34 |
-0.20 |
Southeast Riverine West |
113 |
68 |
-0.13 |
Central Lakes Deciduous East |
82 |
29 |
-0.09 |
Central Lakes Deciduous South |
94 |
47 |
0.12 |
Central Lakes Deciduous West |
62 |
38 |
0.01 |
Central Lakes Coniferous South and East |
114 |
96 |
0.32 |
Central Lakes Coniferous Central, North, and West |
65 |
28 |
0.26 |
Red River Valley |
36 |
45 |
-0.05 |
Northern Bog |
2 |
25 |
-0.02 |
Border Lakes |
36 |
23 |
-0.06 |
Lake Superior |
5 |
63 |
N.A. |
Mean |
58 |
48 |
0.11 |
8.6.2.1 Improvement over Phase 1 models
To measure the improvement of Phase 2 models over Phase 1 models, the Phase 1 models were extended to other counties in the Phase 2 model subregions of which they are a part (Table 8.6.5). For this analysis, Phase 1 models were classified into three probability classes following the same procedures used in the Phase 2 modeling (Section 7.5.1.2).
In these fourteen subregions, the gain statistic improved an average of 0.29 from Phase 1 to Phase 2. This improvement is attributable to several factors. Most important, the variable selection procedure in S-Plus allows consideration of all the variables in the dataset at once. Most Phase 1 models were developed using only logistic regression in GRID, which takes only a small number of variables at once. For those models, variables had to be grouped subjectively for evaluation. The only model from Phase 1 that performs better for a subregion than the Phase 2 model was in Central Lakes Coniferous South. This is the only Phase 1 model that was developed using variable selection in S-Plus. Additional factors contributing to model improvement are the increase in the number of sites available for modeling, the addition of vegetation data from Marschner, and the modeling of subregions, which in some regions reduces the environmental diversity being considered.
Table 8.6.5. Evaluation of Best Phase 1 Model vs. Best Phase 2 Basic Model (excluding single artifacts).
Modeling Region |
Phase 1 Model |
Phase 2 Model |
||||
% Area H/M |
% Sites Predicted |
Gain |
% Area H/M |
% Sites Predicted |
Gain |
|
Southwest Riverine |
45 |
83 |
0.46 |
35 |
86 |
0.59 |
Prairie Lakes East |
60 |
82 |
0.27 |
25 |
64 |
0.61 |
Prairie Lakes North |
63 |
84 |
0.25 |
20 |
71 |
0.71 |
Prairie Lakes South |
59 |
85 |
0.31 |
20 |
77 |
0.74 |
Prairie Lakes East |
52 |
83 |
0.38 |
34 |
72 |
0.53 |
Southeast Riverine West |
67 |
86 |
0.22 |
63 |
87 |
0.28 |
Central Lakes Deciduous East |
67 |
86 |
0.22 |
18 |
82 |
0.78 |
Central Lakes Deciduous South |
47 |
73 |
0.36 |
24 |
81 |
0.70 |
Central Lakes Deciduous West |
76 |
87 |
0.13 |
18 |
81 |
0.78 |
Central Lakes Coniferous West |
41 |
83 |
0.51 |
18 |
76 |
0.76 |
Central Lakes Coniferous East |
77 |
89 |
0.51 |
15 |
82 |
0.82 |
Central Lakes Coniferous North |
39 |
85 |
0.54 |
19 |
84 |
0.77 |
Central Lakes Coniferous South |
35 |
91 |
0.62 |
15 |
25 |
0.40 |
Central Lakes Coniferous West |
49 |
85 |
0.42 |
19 |
63 |
0.70 |
Average |
55.5 |
84 |
0.37 |
24.5 |
74 |
0.66 |
H/M = High/Medium
8.6.2.2 Contributions of Individual Variables
With square root, sine, and cosine transformations included, a total of 120 basic variables were evaluated in each Phase 2 model run (Table 8.6.6). Two additional variables (distance to nearest bedrock outcrop and the square root of the same) were evaluated in the two Southeast Riverine subregions. Considerable redundancy was contributed to the data by slightly different versions of some environmental characteristics (i.e. distance to lakes, distance to large lakes, distance to permanent lakes) and by transformations (square root, sine, cosine) of most variables.
The variable probabilities reported by S-Plus are the best measure for comparison of the performance of individual variables (Section 8.3). Of the 122 variables evaluated in 30 models, all but 13 were assigned a probability greater than zero in at least one model (Table 8.6.6). Fifty-six variables had a maximum probability of 100 in at least one of the thirty runs. Thirteen more had maximum probabilities greater than 80. The cumulative probability is provided in Table 8.6.6 as a measure for ranking variables on a statewide basis. This value was obtained by multiplying the number of model runs in which each variable is not zero by the mean probability for that variable. The results were then analyzed to determine which variables performed best.
Several things are apparent from this analysis. First, transformed variables are better predictors than their untransformed counterparts. Second, distances to large, permanent or perennial water bodies are more consistently useful measures than many of the other distance to water variables. Third, any kind of water or wetland may provide protection from fire and other related resources, such as wood for fuel.
The prominent role of topographic variables suggests they are more universal, applying to a broad range of archaeological regions. It could be that since there are fewer of these landscape variables, there is less repetition or redundancy in what they are measuring versus a much larger suite of water-related variables considered for model construction. Moreover, subtle variations in topography may serve as surrogates for other factors, such as landscape scale vegetation patterns, soil drainage, or visibility, which are not adequately represented in the database. The importance and meaning of these and other variables can only be evaluated with further analysis.
Table 8.6.6. Performance of All Phase 2 Basic Variables in 30 Model Runs.
Columns indicate the number of models in which each variable had probability greater than zero, the maximum probability recorded, and the cumulative probability.
BASIC VARIABLE |
Number of Models |
Maximum Probability |
Cumulative Probability |
Elevation |
8 |
100 |
676.16 |
On alluvium |
1 |
0.8 |
0.8 |
Prevailing orientation |
2 |
87.5 |
186.2 |
On colluvium |
0 |
0 |
|
Distance to well-drained soils |
1 |
60.9 |
60.9 |
Square root of distance to well-drained soils |
2 |
4.7 |
13.8 |
Distance to edge of nearest large lake |
5 |
100 |
492.5 |
Square root of distance to edge of nearest large lake |
10 |
100 |
859 |
Distance to edge of nearest large area of organic soils |
3 |
97.9 |
153 |
Square root of distance to edge of nearest large area of organic soils |
4 |
100 |
257.6 |
Distance to edge of nearest large wetland |
5 |
96.6 |
213 |
Square root of distance to edge of nearest large wetland |
5 |
100 |
311.5 |
Distance to edge of nearest lake, wetland, or area of organic soils |
3 |
22.8 |
34.2 |
Square root of distance to edge of nearest lake, wetland, or area of organic soils |
4 |
59.2 |
98 |
Distance to edge of nearest lake, wetland, area of organic soils, or stream |
4 |
100 |
146 |
Square root of distance to edge of nearest lake, wetland, area of organic soils, or stream |
1 |
18.1 |
18.1 |
Distance to edge of nearest lake |
5 |
78.6 |
132.5 |
Square root of distance to edge of nearest lake |
5 |
93.1 |
233.5 |
Distance to edge of nearest marsh |
3 |
20.1 |
34.8 |
Square root of distance to edge of nearest marsh |
2 |
9.3 |
13.2 |
Distance to edge of nearest large river |
3 |
38.7 |
56.7 |
Square rot of distance to edge of nearest large river |
2 |
100 |
200 |
Distance to edge of nearest area of organic soils |
5 |
100 |
426 |
Square root of distance to edge of nearest area of organic soils |
5 |
100 |
297.5 |
Distance to edge of nearest permanent lake |
9 |
100 |
594 |
Square root of distance to edge of nearest permanent lake |
9 |
100 |
603.9 |
Distance to edge of nearest perennial river or stream |
5 |
100 |
317.5 |
Square root of distance to edge of nearest perennial river or stream |
9 |
100 |
856.8 |
Distance to edge of nearest river or stream |
3 |
100 |
135.9 |
Square root of distance to edge of nearest river or stream |
0 |
0 |
|
Distance to edge of nearest swamp |
1 |
2.9 |
2.9 |
Square root of distance to edge of nearest swamp |
4 |
100 |
233.6 |
Distance to edge of nearest wetland |
4 |
79.7 |
95.2 |
Square root of distance to edge of nearest wetland |
3 |
9.4 |
21.6 |
Depth to bedrock |
3 |
94.5 |
184.5 |
Distance to nearest intermittent stream |
3 |
100 |
138.6 |
Square root of distance to nearest intermittent stream |
4 |
100 |
244.8 |
Direction to nearest permanent water |
2 |
66.6 |
69 |
Sine of direction to nearest permanent water |
1 |
39.3 |
39.3 |
Cosine of direction to nearest permanent water |
1 |
28 |
28 |
Direction to nearest water |
1 |
33.2 |
33.2 |
Sine of direction to nearest water |
1 |
48.2 |
48.2 |
Cosine of direction to nearest water |
2 |
24.8 |
27.8 |
Direction to nearest water or wetland |
6 |
100 |
532.8 |
Sine of direction to nearest water or wetland |
8 |
100 |
703.2 |
Cosine of direction to nearest water or wetland |
2 |
100 |
200 |
Distance to bedrock outcrops |
1 |
13.7 |
13.7 |
Square root of distance to bedrock outcrops |
1 |
3.5 |
3.5 |
Distance to aspen-birch |
2 |
100 |
200 |
Square root of distance to aspen-birch |
3 |
100 |
293.4 |
Distance to birch |
2 |
1.5 |
1.9 |
Square root of distance to birch |
1 |
100 |
100 |
Distance to brushland |
1 |
100 |
100 |
Square root of distance to brushland |
0 |
0 |
|
Distance to Big Woods |
2 |
91.8 |
103.6 |
Square root of distance to Big Woods |
2 |
11.8 |
23.6 |
Distance to conifers |
0 |
0 |
|
Square root of distance to conifers |
3 |
100 |
215.4 |
Distance to cranberry |
2 |
100 |
101.6 |
Square root of distance to cranberry |
2 |
41.5 |
45 |
Distance to hardwoods |
6 |
100 |
361.8 |
Square root of distance to hardwoods |
5 |
100 |
149.5 |
Distance to Kentucky coffee tree |
2 |
43.7 |
53.8 |
Square root of distance to Kentucky coffee tree |
1 |
9.1 |
9.1 |
Distance to glacial lake sediments |
8 |
100 |
286.4 |
Square root of distance to glacial lake sediments |
5 |
100 |
356 |
Distance to sugar maple |
5 |
100 |
324.5 |
Square root of distance to sugar maple |
5 |
100 |
185 |
Distance to mixed hardwoods and conifers |
3 |
99.4 |
116.1 |
Square root of distance to mixed hardwoods and conifers |
3 |
100 |
204.3 |
Distance to oak woodland |
0 |
0 |
|
Square root of distance to oak woodland |
1 |
100 |
100 |
Distance to pine barrens or flats |
4 |
100 |
258.4 |
Square root of distance to pine barrens or flats |
1 |
4.7 |
4.7 |
Distance to pine groves |
1 |
4.3 |
4.3 |
Square root of distance to pine groves |
2 |
7.9 |
10.6 |
Distance to prairie |
2 |
11.2 |
20.4 |
Square root of distance to prairie |
4 |
100 |
296 |
Distance to river bottom forest |
7 |
100 |
510.3 |
Square root of distance to river bottom forest |
4 |
100 |
293.6 |
Distance to woodland |
0 |
0 |
|
Square root of distance to woodland |
1 |
2.8 |
2.8 |
Distance to nearest perennial stream |
2 |
100 |
103.4 |
Square root of distance to nearest perennial stream |
4 |
100 |
335.6 |
Soil drainage |
0 |
0 |
|
Height above surroundings |
7 |
100 |
511 |
Square root of height above surroundings |
10 |
100 |
812 |
Distance to nearest lake or wetland inlet/outlet |
1 |
3.5 |
3.5 |
Square root of distance to nearest lake or wetland inlet/outlet |
2 |
11.6 |
13.6 |
Solar insolation |
0 |
0 |
|
Distance to nearest lake inlet/outlet |
2 |
11.8 |
17.6 |
Square root of distance to nearest lake inlet/outlet |
1 |
81.9 |
81.9 |
On glacial lake sediment |
1 |
37.6 |
37.6 |
Size of nearest lake |
2 |
95.8 |
101.4 |
Square root of size of nearest lake |
2 |
94.5 |
131.8 |
Distance to nearest permanent lake inlet/outlet |
3 |
100 |
237.3 |
Square root of distance to nearest permanent lake inlet/outlet |
6 |
100 |
531.6 |
On mine pits or dumps |
0 |
0 |
|
Vegetation diversity within 0.5 km |
2 |
82.5 |
93.4 |
Vegetation diversity within 1 km |
3 |
100 |
223.8 |
On peat |
0 |
0 |
|
Distance to nearest confluence between perennial streams and large rivers |
2 |
100 |
104.4 |
Size of nearest permanent lake |
4 |
100 |
244 |
Square root of size of nearest permanent lake |
4 |
64.1 |
217.6 |
Relative elevation |
7 |
100 |
403.9 |
Square root of relative elevation |
12 |
100 |
538.8 |
Surface roughness |
6 |
100 |
532.8 |
Distance to nearest confluence between perennial or intermittent streams and large rivers |
3 |
94.9 |
122.7 |
Square root of distance to nearest confluence between perennial or intermittent streams and large rivers |
3 |
100 |
294.9 |
Susceptibility to sedimentation |
0 |
0 |
|
Slope |
5 |
100 |
444.5 |
Square root of slope |
3 |
95.4 |
157.2 |
Distance to nearest confluence between streams of different classes |
2 |
6.6 |
12.6 |
Square root of distance to nearest confluence between streams of different classes |
0 |
0 |
|
On a river terrace |
3 |
100 |
186.9 |
Vertical distance to water |
2 |
100 |
105.6 |
Vertical distance to permanent water |
4 |
100 |
144.4 |
Susceptibility to erosion by water |
0 |
0 |
|
Distance to nearest wetland inlet/outlet |
3 |
41.5 |
45.6 |
Square root of distance to nearest wetland inlet/outlet |
1 |
5.7 |
5.7 |
Distance to nearest permanent wetland inlet/outlet |
5 |
100 |
252 |
Square root of distance to nearest permanent wetland inlet/outlet |
3 |
10.7 |
23.4 |
Contributions of Trygg Variables
Variables derived from Trygg maps were not strong contributors to the 18 Trygg enhanced models run in Phase 2 (Table 8.6.7). For those that did make significant contributions to models (probabilities greater than 50), four are vegetation variables redundant with information derived from Marschner, although from a higher resolution source scale. Only two significant variables, distance to Native American cultural features and square root of distance to junctures of roads and trails with water and wetlands can be derived only from Trygg map data. Results may be different, however, when Trygg maps can be made available in digital format for the entire state. Both continuous coverage and the opportunity to test all of the variables throughout the state could produce better results.
Table 8.6.7. Performance of All Trygg Variables in 18 Phase 2 Model Runs.
Number of models each variable had probability greater than zero, maximum probability recorded, cumulative probability.
BASIC VARIABLE |
Number of Models |
Maximum Probability |
Cumulative Probability |
Distance from grassland (prairie or meadow) |
1 |
88 |
88 |
Square root of distance from grassland |
1 |
2.6 |
2.6 |
Distance to Native American cultural features |
2 |
100 |
106.4 |
Square root of distance to Native American cultural features |
0 |
||
Distance to roads and trails |
0 |
||
Square root of distance to roads and trails |
0 |
||
Distance from wooded land (except swamp) |
2 |
100 |
117.4 |
Square root of distance from wooded land |
3 |
100 |
111.4 |
Distance to junctures of roads and trails with water and wetland resources |
0 |
||
Square root of distance to junctures of roads and trails with water and wetlands |
1 |
100 |
100 |
Vegetation diversity within 510 meters |
1 |
51.9 |
51.9 |
Vegetation diversity within 990 meters |
0 |
||
Distance to wild rice sites |
0 |
||
Square root of distance to wild rice sites |
0 |
||
Distance to beaver sites |
0 |
||
Square root of distance to beaver sites |
0 |
Contributions of High Resolution Soils Variables
Variables derived from high resolution soils data (digital county soil surveys) were used to enhance 14 Phase 2 model runs. Only three of these variables (mean soil reaction [pH] for the surface layer, square root of distance to edge of nearest hydric soils, square root of distance to edge of nearest large area of hydric soils) showed promise (Table 8.6.8). Like Trygg variables, soil variables may perform better when more extensive and continuous coverage is available. Improvements in the spatial accuracy of many of the digital soils surveys may also help.
Table 8.6.8. Performance of All High Resolution Soils Variables in 14 Phase 2 Model Runs.
Number of models each variable had probability greater than zero, maximum probability recorded, cumulative probability.
SOILS VARIABLE |
Number of Models |
Maximum Probability |
Cumulative Probability |
Suitability of soil for archaeological sites, based on soil texture classes |
0 |
||
Mean depth to the lower boundary of the surface layer |
0 |
||
Mean value for clay content of the surface layer |
1 |
2.4 |
2.4 |
Mean value for the available water capacity for the surface layer |
2 |
6.5 |
7.6 |
Mean value for organic matter content of the surface layer |
1 |
1.6 |
1.6 |
Mean soil reaction (pH) for the surface layer |
2 |
100 |
200 |
Mean permeability rate of the surface layer |
2 |
2.9 |
5.7 |
Distance to edge of nearest hydric soils |
0 |
||
Square root of distance to edge of nearest hydric soils |
1 |
69.5 |
69.5 |
Distance to edge of nearest large area of hydric soils |
3 |
42.9 |
49.6 |
Square root of distance to edge of nearest large area of hydric soils |
4 |
100 |
114.6 |
Distance to edge of nearest water (lakes, wetlands, or hydric soils) |
0 |
||
Square root of distance to edge of nearest water (lakes, wetlands, or hydric soils) |
0 |
||
Distance to edge of nearest water (lakes, wetlands, hydric soils, or streams) |
0 |
||
Square root of distance to edge of nearest water (lakes, wetlands, hydric soils, or streams) |
0 |
The Sparse Population Problem
Once the models have been classified into 20, then three, probability classes, the raw model values are disguised. When the regression equation is applied to a region, it produces a value for each cell, which is the estimated probability of a site occurring in that cell. This value can range from zero to one. The ranges and means of these values vary from region to region (Table 8.6.9).
Table 8.6.9. Raw Model Values for Models Excluding Single Artifacts for Phase 2 Models.
Subregion |
Minimum |
Mean |
Maximum |
Std. Dev. |
1 Southwest Riverine |
0.000 |
0.116 |
0.999 |
0.144 |
2e Prairie Lakes East |
0.000 |
0.064 |
0.985 |
0.111 |
2n Prairie Lakes North |
0.000 |
0.283 |
1.000 |
0.258 |
2s Prairie Lakes South |
0.000 |
0.445 |
1.000 |
0.276 |
3e Southeast Riverine East |
0.000 |
0.480 |
0.945 |
0.253 |
3w Southeast Riverine West |
0.003 |
0.812 |
1.000 |
0.122 |
4e Central Lakes Deciduous East |
0.000 |
0.032 |
0.999 |
0.085 |
4s Central Lakes Deciduous South |
0.001 |
0.216 |
0.999 |
0.221 |
4w Central Lakes Deciduous West |
0.000 |
0.023 |
0.998 |
0.060 |
5e Central Lakes Coniferous East |
0.000 |
0.216 |
1.000 |
0.251 |
5s Central Lakes Coniferous South |
0.000 |
0.154 |
0.996 |
0.212 |
5c Central Lakes Coniferous Central |
0.000 |
0.167 |
1.000 |
0.240 |
5n Central Lakes Coniferous North |
0.000 |
0.065 |
0.993 |
0.144 |
5w Central Lakes Coniferous West |
0.000 |
0.111 |
0.999 |
0.168 |
6n Red River Valley North |
0.000 |
0.160 |
0.999 |
0.176 |
6s Red River Valley South |
0.002 |
0.237 |
1.000 |
0.249 |
7e Northern Bog East |
0.009 |
0.448 |
0.745 |
0.226 |
7w Northern Bog West |
0.001 |
0.392 |
0.745 |
0.241 |
8 Border Lakes |
0.000 |
0.089 |
1.000 |
0.185 |
9n Lake Superior North |
0.001 |
0.258 |
0.937 |
0.280 |
9s Lake Superior South |
0.000 |
0.138 |
0.937 |
0.223 |
Comparisons of these values provide a rough indication of the relative probability of finding sites within subregions. Mean values, for models excluding single artifacts, range from 0.023 in Central Lakes Deciduous West to 0.812 in Southeast Riverine West. This high value is an outlier and may indicate problems with the model. For models excluding both single artifacts and lithic scatters, means ranged from 0.051 in Central Lakes Coniferous South to 0.599 in Central Lakes Deciduous South (Table 8.6.10). These values should be interpreted with caution. They may reflect the amount of survey that has occurred in these regions, as well as the potential for sites. For less surveyed regions, values may be lower than would be the case based on true site potential. On the other hand, the biased nature of survey locations may result in values higher than true site potential.
Table 8.6.10. Raw Model Values for Models Excluding Single Artifacts and Lithic Scatters for Phase 2 Models.
Subregion |
Minimum |
Mean |
Maximum |
Std. Dev. |
1 Southwest Riverine |
0.003 |
0.076 |
0.487 |
0.069 |
2e Prairie Lakes East |
0.000 |
0.061 |
0.508 |
0.109 |
2n Prairie Lakes North |
0.000 |
0.243 |
1.000 |
0.356 |
3e Southeast Riverine East |
0.014 |
0.322 |
0.913 |
0.258 |
3w Southeast Riverine West |
0.006 |
0.284 |
0.982 |
0.245 |
4e Central Lakes Deciduous East |
0.000 |
0.081 |
1.000 |
0.167 |
4s Central Lakes Deciduous South |
0.000 |
0.599 |
1.000 |
0.309 |
4w Central Lakes Deciduous West |
0.000 |
0.081 |
1.000 |
0.156 |
5e Central Lakes Coniferous East |
0.001 |
0.074 |
1.000 |
0.220 |
5s Central Lakes Coniferous South |
0.001 |
0.051 |
1.000 |
0.184 |
5c Central Lakes Coniferous Central |
0.000 |
0.333 |
1.000 |
0.292 |
5n Central Lakes Coniferous North |
0.000 |
0.224 |
1.000 |
0.247 |
5w Central Lakes Coniferous West |
0.000 |
0.242 |
1.000 |
0.285 |
6n Red River Valley North |
0.000 |
0.222 |
0.996 |
0.245 |
6s Red River Valley South |
0.000 |
0.396 |
0.999 |
0.286 |
7e Northern Bog East |
0.153 |
0.352 |
0.997 |
0.231 |
7w Northern Bog West |
0.177 |
0.371 |
0.998 |
0.237 |
8 Big Lake |
0.000 |
0.113 |
1.000 |
0.239 |
Comparing model probabilities with data from random surveys, it would appear that the models overestimate site potential. Three random surveys were conducted for this project in the summer of 1996. The Wright County survey found sites on seven percent of the locations surveyed. The model for Central Lakes Deciduous South estimates a mean probability of 0.216, or 22 percent. The Cass County survey had a two percent success rate, which the model for Central Lakes Coniferous Central predicts 0.167 or 17 percent. The Wabasha County survey had the greatest success with sites on 22 percent of the locations surveyed. However, the models for the Southeast Riverine Region predict 0.480 (48 percent and 0.812 (81 percent). Aside from the possible effect of survey bias, this apparent discrepancy can be at least partially explained by unmet potential. In other words, there are more suitable habitats for sites than there are sites. The population density of Minnesota was quite low in the precontact period. Therefore, all suitable locations were not occupied. Consequently, not all places that are equally well-suited for sites contain archaeological properties.
8.6.3 Phase 3 Results – Statewide Models
This section summarizes the Phase 3 models on a statewide basis. Except for the last model discussed (Section 8.6.3.4), all of the models discussed here are simply composites of the regional models. Section 8.6.4 provides detailed evaluations of the models for each Phase 3 modeling region.
8.6.3.1 Site Probability Model
The site probability models developed in Phase 3 are the counterparts of models developed in Phases 1 and 2. They predict the potential for finding precontact archaeological resources across the state. Figure 8.5 provides a composite map of these 20 models, showing the statewide pattern of site potential from the best Phase 3 models.
The average model predicts 86.8 percent of modeled sites in 25 percent of the region's land area. The average gain statistic is 0.68, and the average Kappa (stability value) is 0.54 (Table 8.6.11). These values reflect averages from 20 regions of different sizes, however. When the composite model is evaluated, 86 percent of all modeled sites in the state, excluding single artifacts, are predicted in high and medium probability zones that constitute only 22.82 percent of the state's area. This produces an overall gain statistic of 0.73. This composite model well exceeds the project's goals of predicting 85 percent of known sites in 33 percent of the land area (for a gain statistic of 0.61). However, because composites of the preliminary models were not developed, no Kappa statistic was calculated statewide. The composite model performed well in 2001, when it was tested with 977 sites that were not available when the models were developed. The statewide model predicted 76.6 percent of the new sites, producing a gain statistic of 0.72 for the test population. For the combined training and testing populations, the model predicted 85.5 percent of known sites and produced a gain statistic of 0.73.
To evaluate the degree of confidence in these models, one must consider a suite of factors (Table 8.6.11). The Gain Statistic may be the least reliable of these. A reliable model should both predict an adequate number of test sites (85 percent) in a relatively small area (33 percent of the landscape) and be at least somewhat stable (Kappa > 0.5). Models for only four of the 20 regions meet these criteria:
- Agassiz Lowlands
- Aspen Parklands
- Border Lakes
- Chippewa Plains
However, two of these regions, Agassiz Lowlands and Aspen Parklands, have very few surveyed places. In these cases, the site models may not adequately reflect the distribution of archaeological resources within the subsection.
There is no discernable pattern between the number of sites available for modeling and the overall quality of a model, as measured here. However, any statistical analysis should be improved by a larger sample size. Marginal results achieved by regions with the highest site numbers (Minnesota River Prairie, Big Woods) may have more to do with the nature of the data than with its quantity. Site location errors may be part of the problem, with enough sites erroneously located in unlikely places that it confuses the detectable pattern. For the same reason, model tests may be inaccurate, as site location errors are known to be present in the test data as well. Another possibility is that site function or temporal use may be confusing the analysis. With large samples, the likelihood of having a sizeable number of sites that are different in some way increases. An analysis of the characteristics of sites not predicted by the models may shed some light on this question.
Table 8.6.11. Site Probability Model Performance for All 20 Regions Modeled in Phase 3.
Modeled Region |
Site Probability Models |
||||||||||
No. of Modeled Sites | Site Frequency 1 | % area Hi/Med | % modeled sites predicted | Modeled Gain | Kappa | No. of Test Sites |
% Test Sites Predicted | Test Gain |
% Test and Modeled Sites Predicted | Combined Test and Model Gain | |
Agassiz Lowlands |
53 |
0.00295 |
19.49 |
86.79 |
0.77543 |
0.55809 |
7 |
85.71 |
0.77258 |
86.67 |
0.77512 |
Anoka Sand Plain |
337 |
0.06599 |
24.56 |
83.98 |
0.70755 |
0.49125 |
38 |
81.58 |
0.69891 |
83.73 |
0.70669 |
Aspen Parklands |
59 |
0.00561 |
22.84 |
83.03 |
0.72492 |
0.68100 |
28 |
96.43 |
0.76316 |
87.36 |
0.73854 |
Big Woods |
637 |
0.07934 |
33.93 |
86.18 |
0.60629 |
0.47874 |
69 |
76.81 |
0.55826 |
85.27 |
0.60209 |
Blufflands |
554 |
0.11336 |
34.35 |
87.01 |
0.60522 |
0.52492 |
51 |
54.90 |
0.37432 |
84.30 |
0.59253 |
Border Lakes |
960 |
0.10278 |
18.88 |
88.54 |
0.78676 |
0.67215 |
154 |
76.62 |
0.75358 |
86.89 |
0.78271 |
Chippewa Plains |
513 |
0.06081 |
26.79 |
85.96 |
0.68834 |
0.62861 |
158 |
78.48 |
0.65864 |
84.20 |
0.68183 |
Coteau Moraines /Inner Coteau |
350 |
0.03152 |
34.76 |
86.00 |
0.59577 |
0.49626 |
41 |
70.73 |
0.50855 |
84.40 |
0.58815 |
Glacial Lake Superior Plain/ North Shore Highlands/ Nashwauk Uplands |
86 |
0.00825 |
22.65 |
84.9 |
0.73322 |
0.31592 |
26 |
80.76 |
0.71984 |
83.94 |
0.73016 |
Hardwood Hills |
470 |
0.02394 |
19.2 |
85.53 |
0.77552 |
0.61453 |
54 |
81.48 |
0.76436 |
85.11 |
0.77441 |
Laurentian Highlands |
120 |
0.06315 |
9.94 |
95 |
0.89537 |
0.15717 |
25 |
84 |
0.86921 |
93.10 |
0.89323 |
Littlefork-Vermilion Uplands |
25 |
0.00438 |
18.32 |
92 |
0.80087 |
0.35445 |
22 |
63.63 |
0.71208 |
78.72 |
0.76728 |
Mille Lacs Uplands |
437 |
0.02786 |
14.37 |
86.72 |
0.83429 |
0.57116 |
63 |
76.19 |
0.81139 |
85.40 |
0.8317 |
Minnesota River Prairie |
969 |
0.03088 |
19.84 |
82.98 |
0.76091 |
0.58827 |
57 |
80.70 |
0.75421 |
82.85 |
0.76052 |
Oak Savanna |
121 |
0.01761 |
20.55 |
91.73 |
0.77597 |
0.51064 |
12 |
50.00 |
0.58900 |
87.97 |
0.76640 |
Pine Moraines & Outwash Plains |
474 |
0.03261 |
19.21 |
86.07 |
0.77681 |
0.78248 |
64 |
67.19 |
0.71409 |
83.83 |
0.77085 |
Red River Prairie |
270 |
0.01469 |
29.9 |
84.82 |
0.64741 |
0.47199 |
58 |
87.93 |
0.65996 |
85.33 |
0.64959 |
Rochester Plateau |
81 |
0.01524 |
51.47 |
85.18 |
0.39575 |
0.44729 |
1 |
100 |
0.48523 |
85.37 |
0.39709 |
St. Croix Moraines And Outwash Plains (Twin Cities Highlands) |
126 |
0.05112 |
48.68 |
86.51 |
0.43729 |
0.99927 |
12 |
100 |
0.51318 |
87.68 |
0.44480 |
St. Louis Moraines/ Tamarack Lowlands |
186 |
0.0165 |
9.76 |
87.64 |
0.88864 |
0.50751 |
33 |
66.67 |
0.85361 |
84.47 |
0.88445 |
AVERAGE BY REGION |
341.45 |
0.03843 |
24.97 |
86.83 |
0.67678 |
0.542585 |
48.65 |
77.99 |
0.67733 |
85.33 |
0.70691 |
STATEWIDE EVALUATION |
6828 |
0.03124 |
22.82 |
86.00 |
0.73465 |
NA |
977 |
76.66 |
0.72304 |
85.56 |
0.73329 |
1 Site frequency is the number of sites per square kilometer within a region.
Improvement over Phase 2
Because different regionalization schemes were used, Phase 2 and 3 models cannot be compared on a regional basis. This discussion is based on the average values for the models in each phase. Phase 3 models performed better than Phase 2 models by every measure except gain (Table 8.6.12). The emphasis in model reclassification in Phase 2 was to maximize gain (Section 7.5.1.2.4), while the emphasis in Phase 3 was to predict as close to 85 percent of known sites possible. With this methodological change, it was expected that Phase 3 models would classify more land areas high/medium potential. Despite this, Phase 3 models reduced the area slightly while increasing the percentage of sites predicted significantly. At the same time, Phase 3 models did not, on average, reduce the gain statistics.
Improvements in model performance can be attributed to much larger populations of known sites for deriving models (Sections 7.3.5.2 and 7.5.1.3), refinements in the classification procedures (Section 7.5.1.3), and reducing the environmental variability with regions by using a different regionalization scheme (Section 4.6.3)
Phase 3 models (Figure 8.5) did not completely eliminate the edge effect between regions observed in the Phase 2 models (Section 4.6.2 and Figure 8.4). However, this effect is absent between some regions and inconspicuous between others, particularly when region boundaries follow hydrologic features. There seems to be a relationship to site numbers, as the effect is most conspicuous where regions with very low site numbers abut regions with higher site numbers (e.g. Aspen Parklands and Red River Prairie).
Table 8.6.12. Comparison of Phase 1, Phase 2 and Phase 3 Site Probability Models. Values are averages of those for the separate regional model evaluations based on combined training and test data.
Model statistic |
Phase 1 |
Phase 2 |
Phase 3 |
Percent area in high/medium probability class |
55.5 |
24 |
23 |
Percent sites predicted |
84 |
77 |
85 |
Gain |
0.37 |
0.68 |
0.71 |
Contributions of Individual Variables
Only 44 variables were used to build models in Phase 3, a considerable reduction from Phase 2 (Section 8.6.2.2). It should be noted again that, in Phase 3, all horizontal distance and size variables were transformed to square roots for modeling and should be compared to the results of the square root equivalents in Phase 2. Likewise, direction was converted to sine.
Of the 44 Phase 3 variables, only one (distance to bedrock used for tools) failed to contribute to any site probability model (Table 8.6.13). Clearly, these variables were a more effective set than that used in Phase 2, when 13 variables (11 percent) failed to contribute to any model. All remaining Phase 3 variables had a maximum probability of 100 in at least one model. On the average, each variable figured into six models. Prevailing orientation, relative elevation, and size of nearest permanent lake each contributed to fewer than three models. Distance to edge of nearest large lake, distance to edge of nearest perennial river or stream, distance to nearest lake, wetland, organic soil, or stream, and height above surroundings each contributed to more than ten models. These four variables also had the highest cumulative probabilities.
Cumulative probabilities are perhaps the best measure of the contribution of the variables to the overall modeling effort. The average cumulative probability for the Phase 3 model variables is 561. The variables with above average cumulative probabilities are evenly spread between three aspects of the environment:
- surface hydrology (direction to nearest water or wetland; distance to edge of nearest swamp; distance to edge of nearest large lake; distance to edge of nearest perennial river or stream; distance to nearest lake, wetland, organic soil, or stream)
- vegetation (distance to sugar maple, distance to hardwoods, distance to prairie, vegetation diversity within 1 km)
- topography (surface roughness; vertical distance to permanent water; elevation; height above surroundings; distance to nearest minor ridge or divide).
These results stress the importance of several components of the environment that are significant to hunter/gatherers:
- topographic position (elevation; height above surroundings; distance to nearest minor ridge or divide). This can mean a variety of things, depending on the context. Height above surroundings is the top ranked variable and could indicate locations on rises in a floodplain, tops of bluffs, or defensible positions with wide viewsheds. Distance to nearest minor ridge or divide, if values are low, may indicate higher positions with views or in close proximity to the divide, which may have been used as travel routes. If the same variable has high values, this may indicate locations in the valley floors.
- proximity to water bodies in general (distance to nearest lake, wetland, organic soil, or stream). This is the second most significant variable in the Phase 3 models.
- proximity to large permanent water features (distance to edge of nearest large lake; distance to edge of nearest perennial river or stream; vertical distance to permanent water).
- location with respect to features that can serve as firebreaks (direction to nearest water or wetland, surface roughness)
- proximity to shelter and firewood (distance to sugar maple, distance to hardwoods, distance to edge of nearest swamp). Even distance to prairie, which tends to have an inverse relationship with site location, is an indicator of the importance of shelter and firewood. The more mesic wooded environments may also provide some protection from fire, while prairies would not.
- proximity to a wide range of ecological resources (vegetation diversity within 1 km). Each vegetation type provides a different set of plant and animal products that can be used for food, clothing, shelter, and for making domestic items like baskets. Having several vegetation types within easy access reduces the expenditure of energy required for acquiring these resources.
Table 8.6.13. Performance of All Phase 3 Variables in the Best Site Probability Models.
Number of models in which each variable occurred with probability greater than zero, maximum probability recorded, mean probability recorded, and cumulative probability.
Variable |
# of Models |
Max Prob |
Mean Prob |
Cumulative Prob |
Direction to nearest water or wetland |
8 |
100 |
95.9 |
767.4 |
Distance to aspen-birch |
4 |
100 |
92.9 |
371.4 |
Distance to bedrock used for tools |
0 |
0 |
0.0 |
0.0 |
Distance to Big Woods |
4 |
100 |
98.0 |
391.8 |
Distance to brushlands |
5 |
100 |
85.3 |
426.3 |
Distance to conifers |
6 |
100 |
79.0 |
474.1 |
Distance to edge of nearest large wetland |
6 |
100 |
91.9 |
551.6 |
Distance to edge of nearest area of organic soils |
4 |
100 |
97.6 |
390.3 |
Distance to edge of nearest large lake |
13 |
100 |
97.1 |
1262.4 |
Distance to edge of nearest perennial river or stream |
13 |
100 |
98.6 |
1281.9 |
Distance to edge of nearest swamp |
8 |
100 |
100.0 |
800.0 |
Distance to glacial lake sediment |
4 |
100 |
96.7 |
386.7 |
Distance to hardwoods |
7 |
100 |
93.1 |
651.4 |
Distance to mixed hardwoods and pine |
4 |
100 |
98.0 |
391.8 |
Distance to nearest confluence between perennial or intermittent streams and large rivers |
4 |
100 |
87.4 |
349.7 |
Distance to nearest intermittent stream |
5 |
100 |
89.0 |
445.2 |
Distance to nearest lake inlet/outlet |
6 |
100 |
93.0 |
557.8 |
Distance to nearest lake, wetland, organic soil, or stream |
14 |
100 |
98.0 |
1371.9 |
Distance to nearest major ridge or divide |
4 |
100 |
100.0 |
400.0 |
Distance to nearest minor ridge or divide |
6 |
100 |
97.8 |
586.6 |
Distance to nearest permanent lake inlet/outlet |
4 |
100 |
85.4 |
341.6 |
Distance to nearest permanent wetland inlet/outlet |
4 |
100 |
91.0 |
363.8 |
Distance to oak woodland |
6 |
100 |
93.3 |
559.8 |
Distance to paper birch |
3 |
100 |
99.9 |
299.7 |
Distance to pine barrens or flats |
3 |
100 |
93.2 |
279.7 |
Distance to prairie |
9 |
100 |
89.7 |
807.3 |
Distance to river bottom forest |
3 |
100 |
88.5 |
265.6 |
Distance to sugar maple |
7 |
100 |
89.2 |
624.1 |
Distance to well-drained soils |
3 |
100 |
100.0 |
300.0 |
Elevation |
10 |
100 |
98.9 |
989.0 |
Height above surroundings |
17 |
100 |
98.4 |
1673.6 |
On alluvium |
3 |
100 |
74.7 |
224.1 |
On river terraces |
4 |
100 |
93.9 |
375.6 |
Prevailing orientation |
1 |
100 |
100.0 |
100.0 |
Relative elevation |
2 |
100 |
88.0 |
176.0 |
Size of major watershed |
6 |
100 |
86.7 |
520.1 |
Size of minor watershed |
4 |
100 |
66.8 |
267.0 |
Size of nearest lake |
5 |
100 |
90.9 |
454.6 |
Size of nearest permanent lake |
2 |
100 |
99.1 |
198.2 |
Slope |
6 |
100 |
92.4 |
554.2 |
Surface roughness |
8 |
100 |
86.5 |
692.3 |
Vegetation diversity within 1 km |
10 |
100 |
89.7 |
897.1 |
Vertical distance to permanent water |
9 |
100 |
100.0 |
900.0 |
Vertical distance to water |
4 |
100 |
97.4 |
389.7 |
8.6.3.2 Survey Probability Model
The survey probability models developed in Mn/Model Phase 3 have no precedent. Their development was prompted by the realization that past surveys in Minnesota have been strongly biased in favor of locations near water and that known sites may be absent from other locations simply because no surveys have been conducted there. The survey probability models were developed as a CRM tool to identify the kinds of landscapes that have not been adequately surveyed in the past. Survey potential (Figure 8.6) should be interpreted as the probability for each cell that its environment is similar to places where surveys have occurred. The gain statistic for these models can be seen as a measure of the degree of survey bias, with more biased survey patterns producing higher gain statistics.
The average survey probability model predicts 85 percent of surveyed places in 43 percent of the region's land area, producing a gain statistic of 0.562463 and a Kappa coefficient of stability of 0.56. This implies that, overall, the models perform significantly better than by chance, leading to the conclusion that surveys in the state exhibit a significant amount of locational bias. This bias is apparent in the composite map of the regional models (Figure 8.6).
Since the majority of past surveys have occurred near water, then the majority of the cells near water have been categorized as high survey potential (Figure 8.6). This is particularly apparent in the four regions with the greatest survey bias, as evidenced by strong to very strong gain statistics (0.7 or greater, Table 8.6.14). These models occur in:
- Border Lakes
- Laurentian Highland
- Littlefork-Vermilion Uplands (combined with Agassiz Lowlands and Aspen Parklands)
- St. Louis Moraines/Tamarack Lowlands
All of these, except the Laurentian Highlands, also have strong Kappa values (>0.5), another indicator of the strength of the survey bias. Bias is not a function of the number of places surveyed, though Littlefork-Vermilion Uplands has had the second lowest number of surveys recorded. Border Lakes has more than ten times as many sites as this region and also shows a very high degree of survey bias, probably because of the kinds of places that are accessible in that region. Whether these regions have lower or higher than average numbers of surveys, their surveys have been confined to a limited set of environmental situations, primarily near lakes and rivers. These regions will require additional surveys in the low survey potential zone to provide a more balanced picture of the distribution of their archaeological resources.
Table 8.6.14. Survey Probability Model Performance for All 20 Regions Modeled in Phase 3.
Modeled Region |
Survey Probability Models |
|||||
Surveyed Places |
Survey Frequency |
% area Hi/Med |
% surveys predicted |
Gain |
Kappa |
|
Agassiz Lowlands |
195 |
0.01087 |
36.38 |
87.17 |
0.58265 |
0.63303 |
Anoka Sand Plain |
1016 |
0.19894 |
54.04 |
84.95 |
0.36386 |
0.55217 |
Aspen Parklands |
722 |
0.06863 |
57.68 |
93.07 |
0.38025 |
0.59768 |
Big Woods |
1993 |
0.24824 |
53.32 |
84.7 |
0.37048 |
0.42355 |
Blufflands |
1363 |
0.27889 |
38.65 |
85.03 |
0.54545 |
0.64309 |
Border Lakes |
2230 |
0.23876 |
18.89 |
82.73 |
0.77167 |
0.72217 |
Chippewa Plains |
1685 |
0.19973 |
40.19 |
84.28 |
0.52314 |
0.52964 |
Coteau Moraines /Inner Coteau |
1002 |
0.09024 |
54.62 |
84.03 |
0.34994 |
0.58797 |
Glacial Lake Superior Plain/ North Shore Highlands/ Nashwauk Uplands |
1100 |
0.10435 |
44 |
83.9 |
0.47557 |
0.63578 |
Hardwood Hills |
1509 |
0.07685 |
57.66 |
86.42 |
0.33279 |
0.31642 |
Laurentian Highlands |
746 |
0.3926 |
14.90 |
84.58 |
0.82384 |
0.40028 |
Littlefork-Vermilion Uplands |
207 |
0.0365 |
20.63 |
88.88 |
0.76788 |
0.70685 |
Mille Lacs Uplands |
1543 |
0.09835 |
33.53 |
84.58 |
0.60357 |
0.62430 |
Minnesota River Prairie |
2667 |
0.08498 |
44.63 |
83.24 |
0.46384 |
0.65697 |
Oak Savanna |
733 |
0.1067 |
39.93 |
87.45 |
0.5434 |
0.49516 |
Pine Moraines & Outwash Plains |
1593 |
0.1096 |
43.21 |
83.99 |
0.48553 |
0.59663 |
Red River Prairie |
1051 |
0.05716 |
44.94 |
84.87 |
0.47048 |
0.53891 |
Rochester Plateau |
615 |
0.11574 |
72.77 |
84.72 |
0.14105 |
0.56311 |
St. Croix Moraines And Outwash Plains (Twin Cities Highlands) |
641 |
0.26005 |
61.78 |
87.05 |
0.29029 |
0.44529 |
St. Louis Moraines/ Tamarack Lowlands |
870 |
0.07718 |
24.37 |
82.18 |
0.70846 |
0.54660 |
AVERAGE BY REGION |
1174.05 |
0.142718 |
42.81 |
85.39 |
0.562463 |
0.56078 |
STATEWIDE EVALUATION |
23443 |
- |
43.29 |
84.67 |
0.488721 |
- |
1Site frequency is the number of surveyed places per square kilometer within a region.
Eight regions represent the least biased surveys, evidenced by low gain statistics (<0.5) and higher Kappa coefficients (>0.5):
- Agassiz Lowlands
- Anoka Sand Plain
- Aspen Parklands
- Blufflands
- Coteau Moraines/Inner Coteau
- Minnesota River Prairi
- Pine Moraines and Outwash Plains
- Red River Prairie
- Rochester Plateau
Even in these regions, however, the proclivity to survey environments near water are evident in the model (Figure 8.6).
Contributions of Individual Variables
All 44 Phase 3 variables contributed to the survey probability models (Table 8.6.15). Moreover, each variable contributed, on average, to eight survey probability models compared to an average of six site probability models per variable. Relative elevation, distance to bedrock used for tools, prevailing orientation, size of minor watershed, and vertical distance to water each contributed to fewer than four models. Distance to edge of nearest large lake, height above surroundings, and distance to nearest lake, wetland, organic soil, or stream each contributed to more than 12 models and had the highest cumulative probabilities.
The higher number of models per variable may be attributable to the larger numbers of variables in the survey probability models. Since the number of variables in an individual model seems to be a function of the number of data points, and there are far more surveyed places than known sites, this result should be expected.
Only two variables failed to achieve a maximum probability of 100 in any model. These were relative elevation and distance to bedrock used for tools. Their maximum probabilities were 76.8 and 88.6 respectively. The average cumulative probability for variables in the survey probability models is 74.7. This high value is also a function of having more variables in each model. The variables with higher than average cumulative probabilities include:
- past and present surface hydrology (distance to nearest lake, wetland, organic soil, or stream; distance to edge of nearest large lake; direction to nearest water or wetland; distance to edge of nearest perennial river or stream; distance to nearest confluence between perennial or intermittent streams and large rivers; distance to nearest permanent lake inlet/outlet; size of nearest permanent lake; distance to edge of nearest area of organic soils; distance to glacial lake sediment)
- vegetation (distance to river bottom forest; distance to hardwoods; distance to aspen-birch, distance to oak woodland; distance to pine barrens or flats; vegetation diversity within 1 km)
- topography (height above surroundings; size of major watershed; distance to nearest major ridge or divide; elevation; distance to nearest minor ridge or divide)
These variables are more heavily weighted towards water features than are those for the site probability models. Presumably, these variables represent Minnesota archaeologists' mental models of where sites are likely to be found. These models appear to emphasize:
- proximity to water, particularly large lakes (distance to nearest lake, wetland, organic soil, or stream; distance to edge of nearest large lake; distance to edge of nearest perennial river or stream; size of nearest permanent lake).
- topographic position (height above surroundings; size of major watershed; distance to nearest major ridge or divide; elevation; distance to nearest minor ridge or divide). Field archaeologists tend to focus surveys on higher places in the local landscape (small rises on floodplains, tops of bluffs, positions with a wide viewshed). The role of watershed size is not clear, though it may be a function of the large numbers of surveys conducted along major rivers within the state.
- proximity to sources of shelter and firewood (distance to river bottom forest; distance to hardwoods; distance to aspen-birch, distance to oak woodland; distance to pine barrens or flats). It is difficult to say whether these features were directly selected by archaeologists, by reference to Marschner (1974), for locating surveys or whether they were selected by the modeling process because of their relationships with other variables. For example, the deliberate decision to survey valley bottoms and bluff tops could indirectly result in distance to river bottom forest becoming a significant variable. Interviews with archaeologists about their mental models may clarify the role of these variables.
- protection from fire (direction to nearest water or wetland). Although archaeologists are aware that sites tend to be found on the protected northern and eastern sides of lakes, their mental models apparently do not consider the effectiveness of rolling or steep terrain to slow or stop the spread of fire.
- proximity to features providing evidence of former water bodies (distance to edge of nearest area of organic soils; distance to glacial lake sediment). Glacial lake sediment and organic soils are both evidence of the location and extent of water bodies before the present. Although these features appear to be important to archaeologists, they do not appear as prominently in the site probability models. This is probably explained by the proclivity of archaeologists to look for older, more significant sites. Since these sites are rare, however, they make up only a very small portion of the archaeological database. Consequently, any environmental factors associated with their presence have little significance in the site probability models.
- an emphasis on looking for sites near stream confluences and locations where streams enter lakes or wetlands (distance to nearest confluence between perennial or intermittent streams and large rivers; distance to nearest permanent lake inlet/outlet). These features may be interpreted as nodes on transportation networks. Lake and wetland inlets and outlets may also be locations of wild rice beds, an important seasonal food source.
- proximity to a wide range of ecological resources (vegetation diversity within 1 km). Like the other vegetation variables, it is unclear whether this variable has been used deliberately to locate surveys or whether it is simply correlated with other variables, such as terrain or proximity to water, that are more explicit components of the archaeologists' mental models.
Table 8.6.15. Performance of all Phase 3 Variables in the Best Survey Probability Models.
Number of models where each variable had probability greater than zero, the maximum probability recorded, mean probability, and cumulative probability.
Variable |
# of Models |
Max Prob |
Mean Prob |
Cumulative Prob |
Direction to nearest water or wetland |
11 |
100 |
99.9 |
1098.6 |
Distance to aspen-birch |
10 |
100 |
100.0 |
1000.0 |
Distance to bedrock used for tools |
2 |
88.6 |
84.8 |
169.6 |
Distance to Big Woods |
6 |
100 |
97.0 |
581.8 |
Distance to brushlands |
4 |
100 |
88.1 |
352.4 |
Distance to conifers |
7 |
100 |
82.1 |
574.5 |
Distance to edge of nearest large wetland |
8 |
100 |
90.7 |
725.8 |
Distance to edge of nearest area of organic soils |
11 |
100 |
98.4 |
1082.9 |
Distance to edge of nearest large lake |
14 |
100 |
97.5 |
1365.6 |
Distance to edge of nearest perennial river or stream |
12 |
100 |
86.7 |
1039.8 |
Distance to edge of nearest swamp |
6 |
100 |
100.0 |
600.0 |
Distance to glacial lake sediment |
11 |
100 |
99.9 |
1098.4 |
Distance to hardwoods |
12 |
100 |
94.2 |
1130.3 |
Distance to mixed hardwoods and pine |
6 |
100 |
98.8 |
592.7 |
Distance to nearest confluence between perennial or intermittent streams and large rivers |
10 |
100 |
99.6 |
995.8 |
Distance to nearest intermittent stream |
9 |
100 |
78.3 |
704.7 |
Distance to nearest lake inlet/outlet |
10 |
100 |
99.5 |
994.6 |
Distance to nearest lake, wetland, organic soil, or stream |
17 |
100 |
95.7 |
1626.2 |
Distance to nearest major ridge or divide |
10 |
100 |
98.5 |
985.2 |
Distance to nearest minor ridge or divide |
10 |
100 |
80.1 |
800.7 |
Distance to nearest permanent lake inlet/outlet |
7 |
100 |
99.8 |
698.5 |
Distance to nearest permanent wetland inlet/outlet |
10 |
100 |
98.2 |
982.4 |
Distance to oak woodland |
9 |
100 |
100.0 |
900.0 |
Distance to paper birch |
4 |
100 |
94.9 |
379.5 |
Distance to pine barrens or flats |
8 |
100 |
94.2 |
753.5 |
Distance to prairie |
6 |
100 |
93.6 |
561.8 |
Distance to river bottom forest |
12 |
100 |
98.2 |
1178.2 |
Distance to sugar maple |
5 |
100 |
98.2 |
491.0 |
Distance to well-drained soils |
5 |
100 |
90.3 |
451.7 |
Elevation |
9 |
100 |
97.6 |
878.3 |
Height above surroundings |
14 |
100 |
98.2 |
1375.0 |
On alluvium |
4 |
100 |
89.1 |
356.5 |
On river terraces |
8 |
100 |
89.7 |
717.7 |
Prevailing orientation |
3 |
100 |
89.6 |
268.9 |
Relative elevation |
2 |
76.8 |
71.2 |
142.4 |
Size of major watershed |
12 |
100 |
99.3 |
1191.8 |
Size of minor watershed |
3 |
100 |
99.6 |
298.9 |
Size of nearest lake |
5 |
100 |
75.2 |
375.8 |
Size of nearest permanent lake |
8 |
100 |
95.3 |
762.7 |
Slope |
4 |
100 |
98.7 |
394.8 |
Surface roughness |
4 |
100 |
100.0 |
400.0 |
Vegetation diversity within 1 km |
9 |
100 |
92.1 |
829.2 |
Vertical distance to permanent water |
7 |
100 |
99.9 |
699.5 |
Vertical distance to water |
3 |
100 |
80.8 |
242.4 |
8.6.3.3 Survey Implementation Model
The survey implementation model is an overlay and reclassification of the site probability and survey probability models (Section 7.5.1.3 and Table 7.9). Its primary feature is that it shows areas that have both a low site potential and a low survey potential as being unknown. In this zone, it is likely that site potential is low primarily because these environmental settings have not been adequately surveyed. This zone occupies 49.54 percent of the state's land area and contains five percent of modeled sites and 13 percent of single artifacts (Figure 8.7 and Table 8.6.16). The deficiency of surveys in this region is further highlighted by the low proportions of negative survey points (13 percent) found here. Nearly fifteen percent of test sites were found in the unknown zone, compared to eight percent in the low and possibly low probability zones. This may indicate a somewhat greater emphasis for surveying the unknown areas, as suggested by the Mn/Model implementation plan (Chapter 11).
In regions where the survey probability models are unstable (Table 8.6.14), the unknown area may not be well-identified and may in reality include portions of areas classified as low and possibly low potential. Likewise, unstable site probability models (Table 8.6.11) may have similar effects. Consequently, Kappa coefficients for the site and survey probability models for each region should be considered when using the implementation models to guide future surveys.
The low site potential areas that coincide with medium and high survey potential are assigned site potential values possibly low and low respectively. These are depicted on the map in two shades of yellow. These zones occupy 24 percent of the state's area and contain seven percent of the modeled sites and 15 percent of the single artifacts. However, 34.5 percent of negative survey points are in these zones.
The patterns for the medium and high site potential zones follow those on the site probability models, with the only difference being that these zones are weighted by survey potential. Sites in the medium probability zones are more likely to be found in areas that have higher survey potential (10 percent of modeled sites in 6.5 percent of the state's land area) ) than in areas with lower potential for surveys (2 percent of modeled sites in 2.5 percent of the state's land area). For comparative purposes, this can be reduced to 1.54 vs. 0.8 percent of sites per each one percent of land area. In the high site potential zones, this discrepancy is even greater. By far the largest group of modeled sites (65 percent) is found in the high site potential zone that also has high survey potential (eight percent of the state's area). Only 7.5 percent of sites in the high site potential zone are found in low and medium survey potential locations, which together occupy 2.25 percent of the state's area. For comparison, consider this to be 8.13 vs. 3.33 percent of sites per each one percent of land area. It is apparent that the models are rather conservative about extending the high probability zone much beyond the well-surveyed parts of the landscape.
The majority of sites (77.34 percent) are found in areas where survey potential was rated as high. These are the low, medium, and high site potential classes in the survey implementation model. Another 10.75 percent of sites occur where survey potential is medium (possibly low, medium, and high site potential classes) and 4.92 percent occur where survey potential is low, but where site potential is medium or high (suspected medium and high site potential classes).
Table 8.6.16. Evaluation of Survey Implementation Model for All 20 Regions Modeled in Phase 3.
Site Potential |
Region (30 meter cells) |
Random Points |
Negative Survey Points |
Single Artifacts |
Modeled Sites |
|||||
# |
% |
# |
% |
# |
% |
# |
% |
# |
% |
|
Unknown |
120,328,861 |
49.54 |
23,020 |
49.20 |
2335 |
14.67 |
92 |
12.76 |
370 |
5.43 |
Possibly Low |
31,063,043 |
12.79 |
6037 |
12.90 |
1771 |
11.13 |
36 |
4.99 |
217 |
3.19 |
Low |
27,046,150 |
11.14 |
5164 |
11.04 |
3567 |
22.41 |
75 |
10.40 |
247 |
3.63 |
Suspected Medium |
6,206,642 |
2.56 |
1230 |
2.63 |
142 |
0.89 |
23 |
3.19 |
155 |
2.28 |
Possibly Medium |
7,935,094 |
3.27 |
1484 |
3.17 |
385 |
2.42 |
23 |
3.19 |
177 |
2.60 |
Medium |
15,917,082 |
6.55 |
2909 |
6.22 |
2280 |
14.33 |
74 |
10.26 |
604 |
8.87 |
Suspected High |
2,208,561 |
0.91 |
478 |
1.02 |
71 |
0.45 |
16 |
2.22 |
180 |
2.64 |
Possibly High |
3,326,216 |
1.34 |
6037 |
12.90 |
204 |
1.28 |
32 |
4.44 |
338 |
4.96 |
High |
19,917,051 |
8.20 |
3859 |
8.25 |
5057 |
31.78 |
348 |
48.27 |
4414 |
64.84 |
Water |
7,722,839 |
3.18 |
1647 |
3.52 |
39 |
0.25 |
0 |
0.00 |
61 |
0.90 |
Steep Slopes |
850,789 |
0.35 |
195 |
0.42 |
49 |
0.31 |
2 |
0.28 |
44 |
0.65 |
Mines |
425,116 |
0.18 |
98 |
0.21 |
14 |
0.09 |
0 |
0.00 |
1 |
0.01 |
Total |
242,887,444 |
100 |
46,792 |
100 |
15,914 |
100 |
721 |
100 |
6808 |
100 |
By excluding the unknown zone from consideration, it is possible to evaluate the performance of the site probability model within the kinds of ecological settings that are most likely to have been adequately surveyed. Table 8.6.17 provides recalculations of the percentages of each category of sample points within these site potential classes. Of the 6441 modeled sites found within this area statewide, 91.15 percent are within the six medium and high site potential classes, which constitute 45.28 percent of the adequately surveyed area. This produces a respectable gain statistic of 0.50324, indicating that the model performs significantly better than by chance alone. However, its poor performance compared to the site probability model (Section 8.6.3.1) may be attributable to survey bias, which results in a low level of distinction between places where sites are found and places where surveys have occurred. When future surveys extend the area that can be modeled as adequately surveyed, presumably a stronger model can be produced.
Table 8.6.17. Evaluation of Site Potential for Survey Implementation Model Outside the Unknown Zone.
Site Potential |
Region (30 meter cells) |
Random Points |
Negative Survey Points |
Single Artifacts |
Modeled Sites |
|||||
# |
% |
# |
% |
# |
% |
# |
% |
# |
% |
|
Possibly Low |
31,063,043 |
25.33 |
6037 |
20.72 |
1771 |
13.04 |
36 |
5.72 |
217 |
3.37 |
Low |
27,046,150 |
22.06 |
5164 |
17.72 |
3567 |
26.27 |
75 |
11.92 |
247 |
3.83 |
Suspected Medium |
6,206,642 |
5.06 |
1230 |
4.22 |
142 |
1.05 |
23 |
3.66 |
155 |
2.41 |
Possibly Medium |
7,935,094 |
6.47 |
1484 |
5.09 |
385 |
2.84 |
23 |
3.66 |
177 |
2.75 |
Medium |
15,917,082 |
12.98 |
2909 |
9.98 |
2280 |
16.79 |
74 |
11.76 |
604 |
9.38 |
Suspected High |
2,208,561 |
1.80 |
478 |
1.64 |
71 |
0.52 |
16 |
2.54 |
180 |
2.80 |
Possibly High |
3,326,216 |
2.71 |
6037 |
20.72 |
204 |
1.50 |
32 |
50.9 |
338 |
5.25 |
High |
19,917,051 |
16.24 |
3859 |
13.24 |
5057 |
37.24 |
348 |
55.33 |
4414 |
68.56 |
Water |
7,722,839 |
6.30 |
1647 |
5.65 |
39 |
0.29 |
0 |
0.00 |
61 |
0.94 |
Steep Slopes |
850,789 |
0.69 |
195 |
0.67 |
49 |
0.36 |
2 |
0.32 |
44 |
0.68 |
Mines |
425,116 |
0.35 |
98 |
0.34 |
14 |
0.10 |
0 |
0.00 |
1 |
0.02 |
Total |
122,618,583 |
100 |
29,138 |
100 |
13,579 |
100 |
629 |
100 |
6438 |
100 |
8.6.3.4 Site Probability Model Developed from Statewide Database
Because of difficulties encountered when trying to produce comparable raw model scores for the 20 regional models, a single site probability model was developed from the entire statewide database (Section 7.7). To develop this model, a number of the Phase 3 variables had to be excluded because they were not present statewide. These included distance to paper birch, distance to Big Woods, distance to oak woodland, distance to mixed hardwoods and pine, distance to pine barrens or flats, distance to aspen-birch, distance to bedrock used for tools, distance to conifers, distance to nearest permanent wetland inlet/outlet, and distance to prairie. This left a total of 34 variables for modeling, of which 27 contributed to the model (Table 8.6.18). The only variables with less than 100 percent probability were size of nearest lake and size of nearest permanent lake. The large number of model variables is undoubtedly a function of the very large number of sites in the database.
The resulting model emphasizes the gross patterns in known site distribution (Figure 8.8) and does not articulate the local patterns within the landscape as finely as does the statewide model derived using regionalization (Figure 8.4). Consequently, sites that cannot be explained by large-scale environmental patterns or that depend on local variables are not as well predicted as in the regionalized model. However, this model does provide a more accurate representation of relative probabilities statewide (Figure 8.8). The consequence is that some regions (for instance the Twin Cities metro area and the Arrowhead Region) show very high concentrations of high site potential, while others (like the northern tier of counties west of the Arrowhead) show only limited occurrences of high site potential. Whether these results are interpreted as indicators of ecological settings preferred by hunter-gatherers or as artifacts of past survey efforts, this model is a useful point of comparison with the regionalized site probability model.
Table 8.6.18. Site Probability Model from Statewide Database.
Variable |
S-Plus Regression Coefficient |
Probability |
Direction to nearest water or wetland (sine) |
-0.2325406 |
100.0 |
Distance to edge of nearest large wetland |
0.006508734 |
100.0 |
Distance to edge of nearest area of organic soils |
0.004957566 |
100.0 |
Distance to edge of nearest large lake |
-0.01192841 |
100.0 |
Distance to edge of nearest perennial river or stream |
-0.01827615 |
100.0 |
Distance to edge of nearest swamp |
0.01325694 |
100.0 |
Distance to hardwoods |
-0.004220171 |
100.0 |
Distance to nearest confluence between perennial or intermittent streams and large rivers |
-0.003289315 |
100.0 |
Distance to nearest intermittent stream |
0.006548222 |
100.0 |
Distance to nearest lake inlet/outlet |
-0.01227073 |
100.0 |
Distance to nearest lake, wetland, organic soil, or stream |
-0.04851173 |
100.0 |
Distance to nearest permanent lake inlet/outlet |
0.005728610 |
100.0 |
Distance to river bottom forest |
0.002051763 |
100.0 |
Distance to sugar maple |
0.003129357 |
100.0 |
Distance to well-drained soils |
-0.005608923 |
100.0 |
Elevation |
0.8425730 |
100.0 |
Height above surroundings |
0.02045504 |
100.0 |
On river terraces |
0.7578046 |
100.0 |
Prevailing orientation |
-0.001215266 |
100.0 |
Relative elevation |
0.02036699 |
100.0 |
Size of major watershed |
-0.00001451567 |
100.0 |
Size of minor watershed |
0.00004488181 |
100.0 |
Size of nearest lake |
-0.00005703235 |
48.5 |
Size of nearest permanent lake |
0.00006991912 |
69.9 |
Surface roughness |
-0.01668705 |
100.0 |
Vegetation diversity within 1 km |
0.3938607 |
100.0 |
Vertical distance to permanent water |
-0.006548323 |
100.0 |
This model predicts 84.87 percent of all modeled sites within the high and medium probability zones, which constitute 33.65 percent of the state's area (Table 8.6.19). This produces a good gain statistic (0.60351), indicating that the model performs well and comes very close to meeting project goals. The site probability model developed as a composite of the regional models (Section 8.6.3.1) performs somewhat better because regionalization makes it possible to discern patterns within individual regions, not just statewide. Consequently, regionalization gives more weight to sites in regions with low site numbers.
This model tested well, predicting 82.81 percent of new sites. The gain statistic for the test population is 0.59365.
Because of time constraints, no preliminary models were run, so no Kappa coefficients could be calculated. Performing this analysis would be very useful, especially as a comparison to the stability patterns within the regional models. Also because of time constraints, no survey probability model was developed statewide. The development of survey probability and survey implementation models from the statewide database would also provide useful information for comparison with the regional models.
Table 8.6.19. Evaluation of Site Probability Model from Statewide Database.
Low |
Medium |
High |
Water |
Steep slopes |
Mines |
Total |
||
Region (30 meter cells) |
# |
152,146,814 |
23,271,112 |
58,469,737 |
7721980 |
850883 |
425122 |
242,885,648 |
% |
62.64 |
9.58 |
24.07 |
3.18 |
0.35 |
0.18 |
100.0 |
|
All random points |
# |
28804 |
4555 |
11501 |
1641 |
191 |
98 |
46790 |
% |
61.56 |
9.73 |
24.58 |
3.51 |
0.41 |
0.21 |
100.0 |
|
All negative survey points |
# |
6356 |
1698 |
7758 |
40 |
46 |
14 |
15912 |
% |
39.94 |
10.67 |
48.76 |
0.25 |
0.29 |
0.09 |
100.0 |
|
All modeled sites |
# |
926 |
500 |
5283 |
61 |
43 |
1 |
6814 |
% |
13.59 |
7.34 |
77.53 |
0.9 |
0.63 |
0.01 |
100.0 |
|
All test sites | # | 155 |
63 |
746 |
10 |
3 |
0 |
977 |
15.86 |
6.45 |
76.36 |
1.02 |
0.31 |
0 |
100.0 |
8.6.4 Phase 3 Results – Regional Models
The following sections report on the regionalized models. Reports are presented for 17 individual ECS subsections and for three sets of combined subsections (Figure 8.1). For six of the individual subsections reported, models are taken from modeling combinations of adjacent subsections, as described in Section 7.5.1.3. Only when two or more subsections share the same best site probability and survey probability models are they reported on in combination.
The regional model reports contain descriptions of the environmental context of the region, descriptions and evaluations of the site probability, survey probability, and survey implementation models for that region, and interpretations of the site probability and survey probability models. The order in which the models are presented is as follows:
- 8.7 Agassiz Lowlands
- 8.8 Anoka Sand Plain
- 8.9 Aspen Parklands
- 8.10 Big Woods
8.11 Blufflands- 8.12 Border Lakes
- 8.13 Chippewa Plains
- 8.14 Coteau Moraines / Inner Coteau
- 8.15 Glacial Lake Superior Plain/Northshore Highlands/ Nashwauk Uplands
- 8.16 Hardwood Hills
- 8.17 Laurentian Highlands
- 8.18 Littlefork-Vermilion Uplands
- 8.19 Mille Lacs Uplands
- 8.20 Minnesota River Prairie
- 8.21 Oak Savanna
- 8.22 Pine Moraines & Outwash Plains
- 8.23 Red River Prairie
- 8.24 Rochester Plateau
- 8.25 St. Croix Moraines and Outwash Plains (Twin Cities Highlands)
- 8.26 St. Louis Moraines/ Tamarack Lowlands
Site probability models developed in Phase 3 of this project performed very well and met or exceeded project goals. However, several factors limit their overall quality. First and foremost, there are simply too few known sites in some parts of the state to impart a high level of confidence to the models for those areas. In some cases (for example, Laurentian Highlands, St. Louis Moraines and Tamarack Lowlands), the site probability models performed deceptively well. This can be explained by the very limited range of variation in the known sites' environments. This limited range of variation, in turn, results from survey bias, low survey numbers, and low site numbers. In such cases, model stability may be rather high. Again, this is deceptive, as the range of variability in the site environment data is too narrow to introduce sufficient uncertainty into the model. Thus, interpretation of all model results presented here should be tempered by the number of sites available for analysis within each subsection.
Similarly, when a large number of sites are taken from biased surveys, as in the Border Lakes subsection, the environmental variability of the site locations may still be low. The survey probability and survey implementation models were developed to address this kind of bias. However, survey bias may have been overestimated in this phase of the project, as surveys are not yet adequately mapped. A more complete mapping of surveys should reduce the amount of land classified as "unknown" in the survey implementation models.
These models may also include errors or inaccuracies attributable to site mapping problems. As only site centroids were mapped for this project, a more limited range of environments may have been analyzed than would have been included in the database if sites were mapped as polygons. Moreover, inaccurate or imprecise centroid locations may have resulted in the inclusion of atypical environments in the analysis. Improved site mapping will be an important component of future models.
Although the edge effect that was so conspicuous in the Phase 2 models (Figure 8.4b) is not as apparent, it can still be detected in the Phase 3 models (Figure 8.5), particularly along the margins of the Aspen Parklands, Oak Savanna, Rochester Plateau, Red River Prairie, and Nashwauk Uplands. These subsections all have low site numbers and low site frequencies. However, this is almost certainly not the entire explanation for the phenomenon. To some extent it may be an artifact of how the raw model values are classified into probability classes, which permits adjacent regions to have larger or smaller proportions of their areas classified as high and medium site probability. Other, sometimes more conspicuous, edge effects are apparent where elevation data of different qualities adjoin.
These models best predict more recent archaeological sites (i.e., those formed within the last 3500 years), as these make up a majority of the available data and will be the most closely associated with modern environmental variables. Combined with the landscape sediment assemblage interpretations (Chapter 12) and hydrologic modeling to identify locations of drained lakes, the site/environment relationships identified by these models may help develop models for earlier archaeological sites via reconstructions of pre-Woodland suitable habitats.
All of these limitations can be addressed in the next full modeling phase, which is scheduled to take place in about 2006-2007. Plans for those future enhancements are discussed in Chapter 10.
Bonham-Carter, G.F.
1994 Geographic Information Systems for Geoscientists: Modeling
with GIS. Pergamon
(Elsevier Science Ltd.), Tarrytown, NY.
Brandt, R., B.J. Groenewoudt, and
K.L. Kvamme
1992 An Experiment in Archaeological Site Location: Modeling in
the Netherlands using GIS
Techniques. World Archaeology 24:268-282.
Carmichael, D.L.
1990 GIS Predictive Modeling of Prehistoric Site Distributions
in Central Montana. In Interpreting
Space: GIS and Archaeology, edited
by K.M.S. Allen, S.W. Green, and E.B.W. Zubrow, pp.
216-225. Taylor and Francis, London.
Cassell, M.S., H.D. Mooers, C.A.
Dobbs, T. Madigan, M. Covill, J. Berry, and D.A. Birk
1997 An Archaeological Sensitivity Model of Prehistoric and
Contact Period Settlement at
Camp Ripley, Morrison County, Minnesota.
Reports of Investigation No. 397. Institute for
Minnesota Archaeology, Minneapolis.
Craig, J.
1989 Predictive Modeling of Prehistoric Settlement Patterns in
the Chicago Lake Plain. The
Wisconsin Archaeologist 70 (3):347-361.
Dalla Bona, L.
1994 Methodological Considerations. Cultural Heritage Resource
Predictive Modeling Project
Vol.4. Centre for Archaeological Resource
Prediction, Lakehead University, Thunder Bay, Ontario.
Dalla Bona, L. and L. Larcombe
1996 Modeling Prehistoric Land Use in Northern Ontario. In New
Methods, Old Problems:
Geographic Information Systems in Modern
Archaeological Research, edited by H.D.G.
Maschner, pp. 252-271. Center for Archaeological
Investigations Occasional Paper No. 23,
Southern Illinois University, Carbondale.
Dobbs, C.A., Breakey, K.C., and
H. Mooers
1994A Model of Archaeological Sensitivity for Landforms along
the Lakehead Pipeline Corridor
from Neche, North Dakota to Clearbrook,
Minnesota. Reports of Investigation No. 282.
Institute for Minnesota Archaeology, Minneapolis.
Dobbs, C.A. and H. Mooers
1990 A Preliminary Model of Archaeological Sensitivity for Landforms
along the Great Lakes Gas
Transmission Company Natural Gas Pipeline
Corridor from St. Vincent Minnesota to Rapid
River, Michigan. Reports of Investigation
No. 96. Institute for Minnesota Archaeology,
Minneapolis.
Grimm, E.C.
1994 Fire and Other Factors Controlling the Big Woods Vegetation
of Minnesota in the Mid-Nineteenth Century. Ecological Monographs 54:291-311.
Hasentrab, R.J.
1991 Wetlands as a Critical Variable in Predictive Modeling of
Prehistoric Site Locations: A Case
Study from the Passaic River Basin. Man
in the Northeast 42:39-61.
Howes, D.
1982 A Predictive Model for Site Location in the Alberta Foothills. Plains Anthropologist 27:97-
108.
Jochim, M.A.
1976 Hunter-Gatherer Subsistence and Settlement: A Predictive
Model. Academic Press, New
York.
Kellogg, D.C.
1987 Statistical Relevance and Site Locational Data. American
Antiquity 52:143-150.
Kohler, T.A.
1988 Predictive Locational Modeling: History and Current Practice.
In Quantifying the Present
and Predicting the Past: Theory, Method,
and Application of Archaeological Predictive
Modeling, edited by W. J. Judge and
L, Sebastian, pp. 19-59. U.S. Government Printing Office,
Washington, DC
Kvamme, K.L.
1985 Determining Empirical Relationships Between the Natural Environment
and Prehistoric Site
Locations: A Hunter-Gatherer Example. In For Concordance in Archaeological Analysis:
Bridging Data Structure, Quantitative Technique,
and Theory, edited by C. Carr, pp. 208-
238. Westport Press, Kansas City.
1988 Development and Testing
of Quantitative Models. In Quantifying the Present and
Predicting the Past: Theory, Method, and
Application of Archaeological Predictive
Modeling, edited by W. J. Judge and
L, Sebastian, pp. 325-428. U.S. Government Printing
Office, Washington, DC
1992 A Predictive Site
Location Model on the High Plains: An Example with an Independent Test.
Plains Anthropologist 37:19-40.
1994 Ranter’s Corner:
GIS graphics vs. spatial statistics: how do they fit together? Archaeological
Computing Newsletter 38:1-2.
Larson, T.K., R.G. Hilman, and J.D.
Benko
1991 Site Patterning Analysis of the Granite Falls Locality. In
pp. The 1990 Archaeological
Investigations at the Seim/Livingood Site,
21 CP 29, Chippewa County, Minnesota, edited by
T.K. Larson, D.M. Penny, and A.R. Woolworth,
pp. 10.1-10.17. Submitted to the Minnesota
Department of Transportation, St. Paul.
Lafferty, R.H., III, S. Parker,
and W.F. Limp
1981 Testing the Sparta Hypotheses. In Model Validation in Sparta,
edited by R.H. Lafferty, III.
And J.H. House, pp.206-229. Research Report
No. 25, Arkansas Archaeological Survey,
Fayetteville.
Larralde, S.L. and S.M. Chandler
1981 Archaeological Inventory in the Seep Ridge Cultural Study
Tract, Uintah County,
Northeastern Utah: With a Regional Predictive
Model for Site Location. Cultural Resources
Series 11, Bureau of Land Management, Salt
Lake City.
Limp. W.F., R.H. Lafferty, III,
S.C. Scholtz
1985 Toward a Model of Location Choice in Sparta. In Settlement
Predictions in Sparta: A
Location Analysis and Cultural Resource
Assessment in the Uplands of Calhoun County, edited
by W.F. Limp, pp. 59-99. Research Series
No. 14, Arkansas Archaeological Survey,
Fayetteville.
Marschner, F.J.
1974 The Original Vegetation of Minnesota. Compiled from
U.S. General Land Office Survey
notes. U.S. Department of Agriculture,
Forest Service, North Central Forest Experiment Station,
St. Paul, Minnesota.
Minnesota DNR (Department of Natural
Resources)
1998 Ecological Classification System, URL: http://www.dnr.state.mn.us/ecs/index.html
Neumann, T.W.
1992 The Physiographic Variables Associated with Prehistoric Site
Location in the Upper Potomac
River basin, West Virginia. Archaeology
of Eastern North America 20:81-124.
Pilgrim, T.
1987 Predicting Archaeological Sites from Environmental Variables:
A Mathematical Model for the
Sierra Nevada Foothills, California.
BAR International Series 320. Oxford, England.
Shermer, S.J. And J.A. Tiffany
1985 Environmental Variables as Factors in Site Location: An Example
from the Upper Midwest.
Midcontinental Journal of Archaeology 10:215-240.
Warren, R.E.
1990 Predictive Modeling of Archaeological Site Location: A Case
Study in the Midwest. In
Interpreting Space: GIS and Archaeology,
edited by K.M.S. Allen, SW Green, and E.B.W.
Zubrow, pp. 201-215. Taylor and Francis,
London.
Warren, RE and D.L. Asch
1996 A Predictive Model of Archaeological Site Location in the
Eastern Prairie Peninsula, Illinois.
Unpublished manuscript, Illinois State
Museum, Springfield.
Williams, L., D.H. Thomas, and R.
Bettinger
1973 Notions to Numbers: Great Basin Settlements as Polythetic
Sets. In Research and Theory in
Current Archeology, edited by C.L.
Redmond, pp. 215-237. John Willey and Sons, New York.
Young, P.M., M.R. Horne, C.D. Varley,
P.J. Racher, and A.J. Clish
1995 A Biophysical Model for Prehistoric Archaeological Sites
in Southern Ontario. Research and
Development Branch, Ministry of Transportation,
Ontario.
The Mn/Model Final Report (Phases 1-3) is available on CD-ROM. Copies may be requested by visiting the contact page.
Acknowledgements
MnModel was financed with Transportation Enhancement and State Planning and Research funds from the Federal Highway Administration and a Minnesota Department of Transportation match.
Copyright Notice
The MnModel process and the predictive models it produced are copyrighted by the Minnesota Department of Transportation (MnDOT), 2000. They may not be used without MnDOT's consent.